🤖 Machine Learning: Heavy Industries Applications 🚜
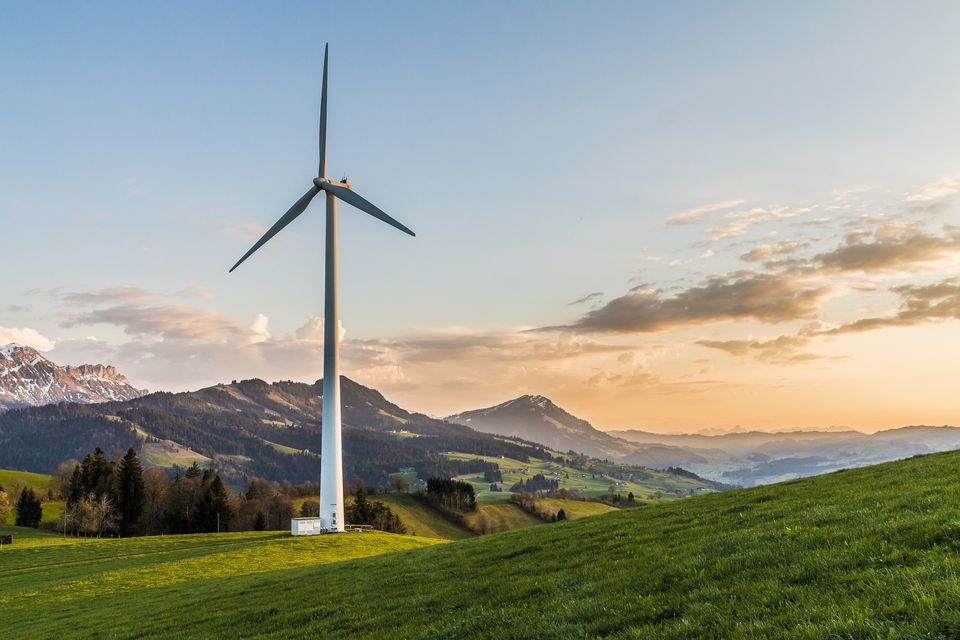
If you ask in the geek atmosphere, it’s very easy to get examples of how Machine Learning is applied in the tech industry. You will get answers like product recommendations, spam filtering, online fraud detections, computer vision, and many others.
However, if you ask the same question about the applications in heavy industries, they definitely have to think about it a little bit to give you an answer.
In addition, we all know Artificial Intelligence and Machine Learning algorithms are everywhere, we are using them while we use Netflix, Apple’s Siri, Amazon’s Alexa, Google’s Waze, or the weather forecasting app.
Therefore, in this article I am going to cover several applications of Artificial Intelligence for heavy industries, in each part, I will also give you examples of actual AI companies delivering that kind of machine learning products.
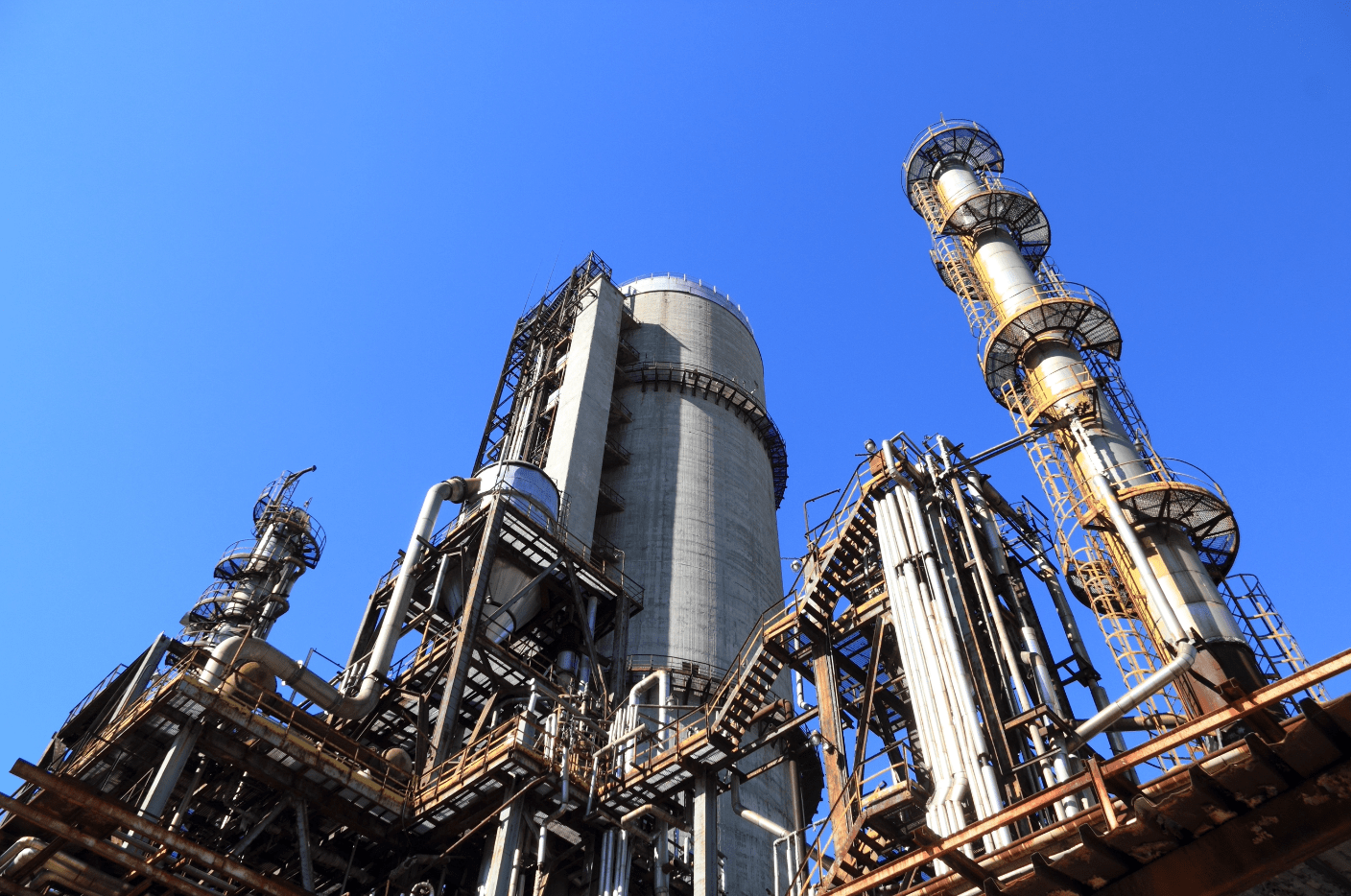
What exactly is a heavy industry?
Generally speaking, is a company that has huge investments in production assets to produce something on a big scale. Examples are oil & gas, electricity, chemicals, cement, mining, steel & metals, and big manufacturing. These industries are also known as capital intesive industries.
Nowadays, capital intensive industries have well implemented digital automation and instrumentation in their processes, so there is a huge opportunity to apply industrial Artificial Intelligence over that data to improve different aspects of their operations.
Therefore, in this article you will know some applications of AI that can be used in heavy industries.
1. Benefits of AI in Heavy Industries
First, I want to enumerate some of the most important benefits of using AI in Heavy Industries, just to point how important this is:
- Improvement of process safety
- Improvement of process availability
- Reduce total energy consumption
- Lower emissions
- Higher margins for the business
- Lower costs
- Low spare parts inventory
- Time to plan maintenance around future failures
- Improvement of operational excellence
- Reduce human expertise dependency
2. AI Applications in Heavy Industries
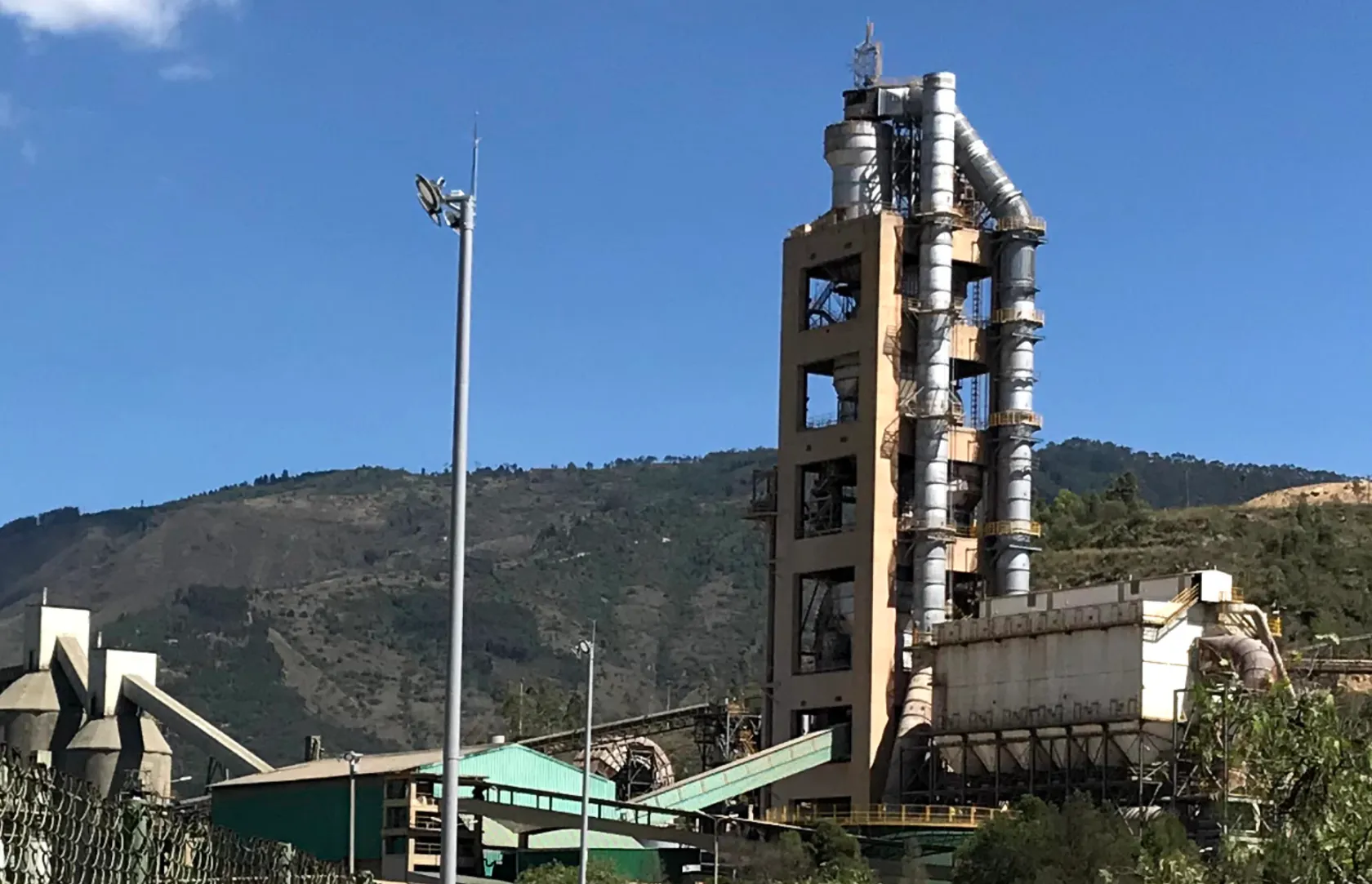
2.1 Predictive Maintenance
For me, this is one of the most amazing and impressive applications of AI in heavy industries. Companies are able to predict failures several days before they occur, depending on the machine could be even months.
Before explaining what is predictive maintenance, it’s very important to point out that every machine eventually brakes down.
Every single part will wear, and from time to time, they have to be repaired or replaced, and with time the whole machine must be changed.
Traditionally, companies base their maintenance schedule in two plans:
- Reactive maintenance plan
- Preventive maintenance plan
Let’s take a look at these concepts.
2.1.1 Reactive Maintenance
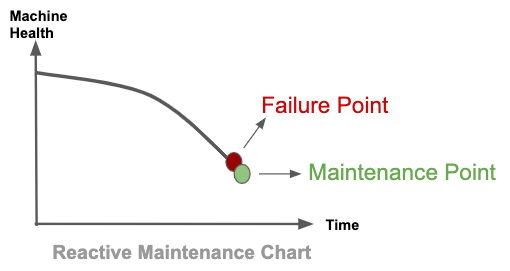
This kind of maintenance consists of the operation of the machine to the point of failure. In the downtime that the failure produces, the engineers start the corrective maintenance procedures and repair the machine.
This is good for a lamp but not for an airplane engine.
2.1.2 Preventive Maintenance
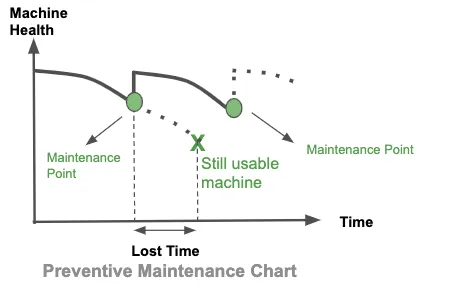
So, to avoid that a plane crashes, maintenance engineers design preventive maintenance cycles on every machine.
Indeed, the goal is to execute the maintenance procedures and parts replacement before the point of failure, so there is no chance that the machine breaks down.
With this approach, the machine downtime is previously known allowing to have a backup plan and control the actual downtime of the plant or machine.
However, the companies are doing maintenance in machines that still have a long time to work without failures, losing a lot of money.
Otherwise, every maintenance engineer in the world knows that preventive maintenance is not enough, so the machines are having problems all the time.
2.1.4 Predictive Maintenance Concept
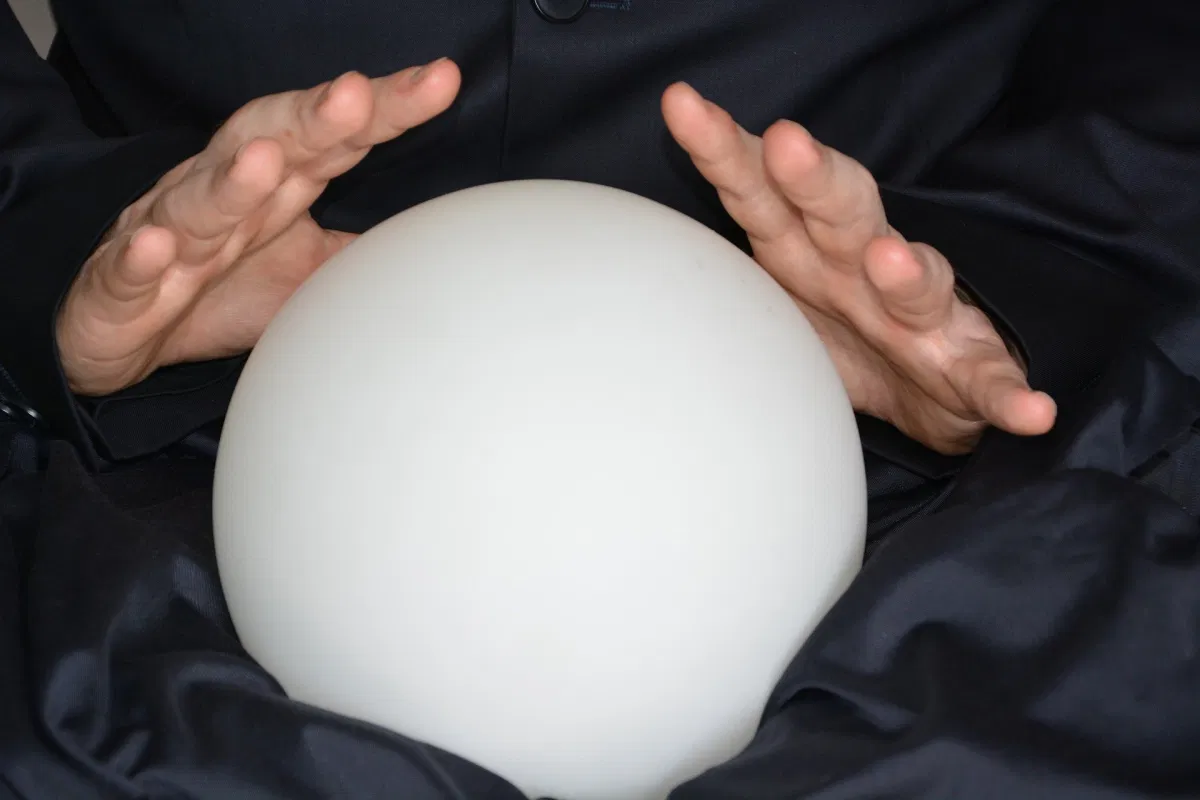
Chemical plants, cement factories, oil&gas facilities, etc. gather and store big amounts of data every second, thanks to having a very well implemented digital automation and instrumentation, almost from the very beginning of these technologies.
As I told you before, this historical data sometimes only remains in a storage server without been used.
Consequently, this big amount of data is GOLD for Data Scientists, and of course for the company, only if they decide to use it wisely.
So, What if it’s possible to predict when the machine will fails using this data?
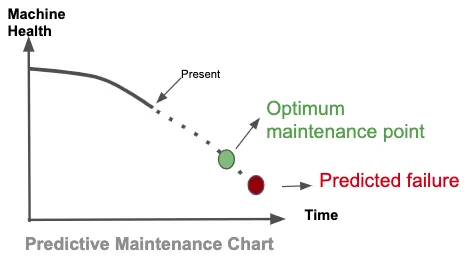
Therefore, if we can predict when the machine or part of it will fail, we can reach the Optimum Maintenance Time, which is having the maintenance date safely close enough to the predicted failure date. This strategy allows us to have a very short Lost Time between maintenance cycles.
Therefore, this becomes in a huge benefits creator for the company that implements it, doing the business more profitable and safer.
Benefits of predictive maintenance:
- Improvement of process safety
- Much less human casualties in maintenance operations
- Improvement of process availability
- Lower emissions due to prevent equipment rupture
- Low spare parts inventory
- Time to plan maintenance around predicted failures
- Save money
- Increase productivity
Nowadays, several industrial Artificial Intelligent companies can implement Machine Learning models for predictive maintenance using Deep Learning Algorithms for anomaly detection in time series.
Some companies offering this product are GE, Aspen Technology, AVEVA, UPTAKE, Arundo Analytics, and others.
2.2 Energy Consumption Optimization
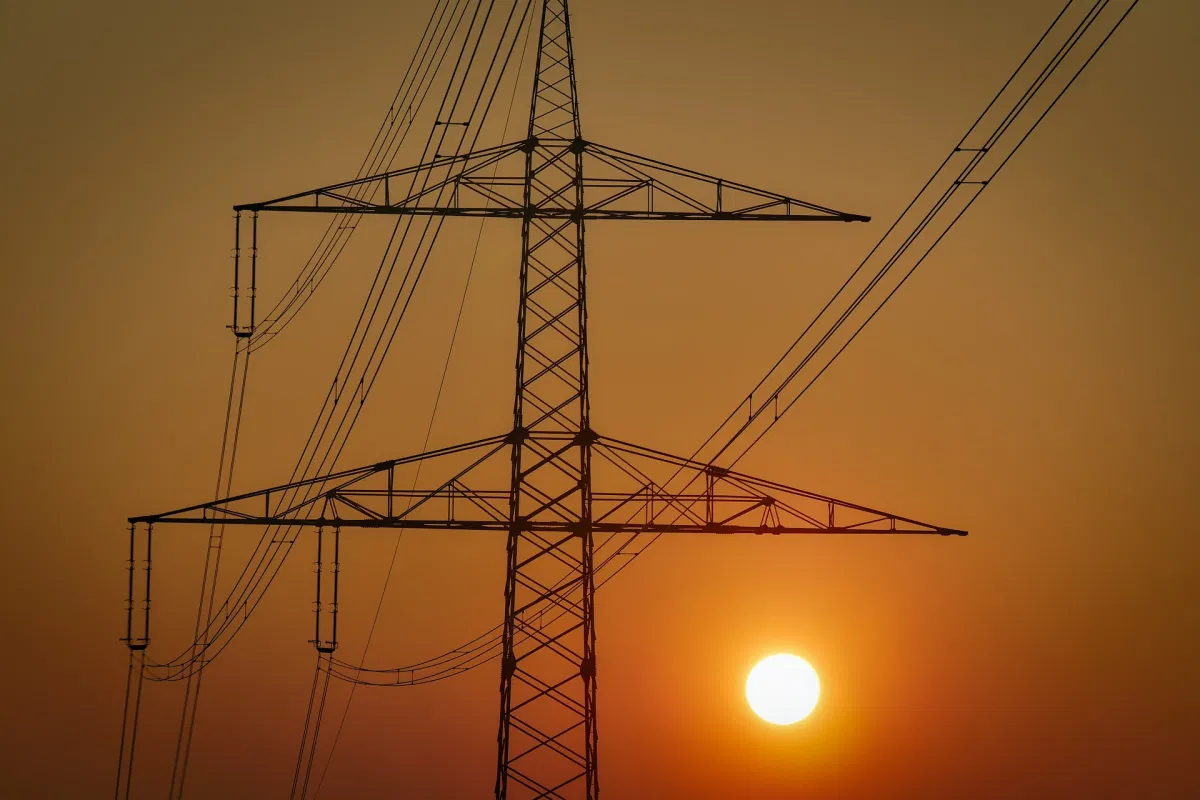
The energy supply is a key factor in every heavy industry, it fuels all the process units and operations, without it, it’s physically impossible to produce anything. Therefore, each company has to guarantee its incoming energy flow at minimum cost, however, achieve this is not an easy task.
Indeed, we can safely say that energy is the most important “raw material” of heavy industry, but it costs, it costs a lot.
This is why companies are always looking to optimize their energy consumption, which in other words, means to consume less oil, less carbon, less natural gas, less electricity.
Also, energy consumption is directly related to greenhouse emissions, if they can reduce energy consumption, they will reduce gas emissions, as simple as that.
So, here there is a good opportunity to apply Artificial Intelligence models to Optimize Energy Consumption.
2.2.1 How is applied AI for Energy Optimization?
In order to model this problem, it’s necessary to have data on energy consumption for many years, of course, if that’s possible. However, the time series of energy used is not enough, you need another kind of data to have a good model to optimize energy in the whole facility.
That kind of data is the energy flow inside the plant, that in simple words represents how the process units exchange energy between them and the environment.
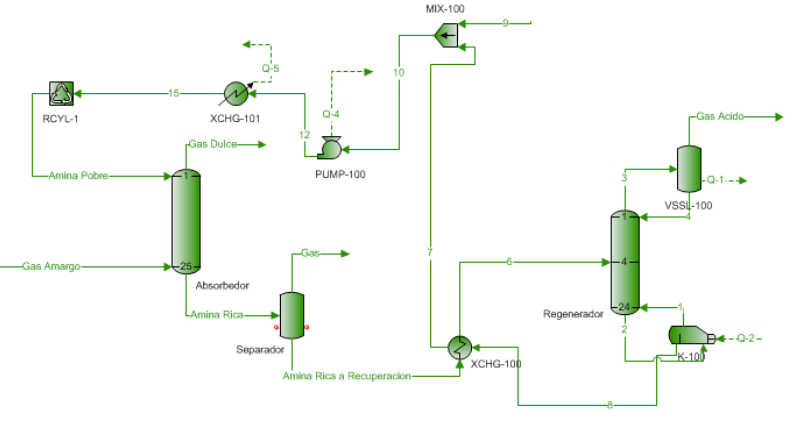
Look at the process diagram above, it has several process units (machines) that exchange materials and energy between them using pipes in order to remove CO2 and H2S from raw natural gas.
In this process, it’s necessary to consume heat for the regenerator reboiler and electricity or compressed air for pumps and instrumentation.
Therefore, with the energy flows of the process and the historical data, it’s possible to train a deep neural network to find optimization points, energy flow patterns, or optimize process parameters in order to reduce energy usage without compromise the process productivity.
Some companies offering this product are  GE, Aspen Technology, UPTAKE, Arundo Analytics, METRON, AVEVA, and others.
2.3 Determination of process parameters: Virtual Sensors - Digital Twins
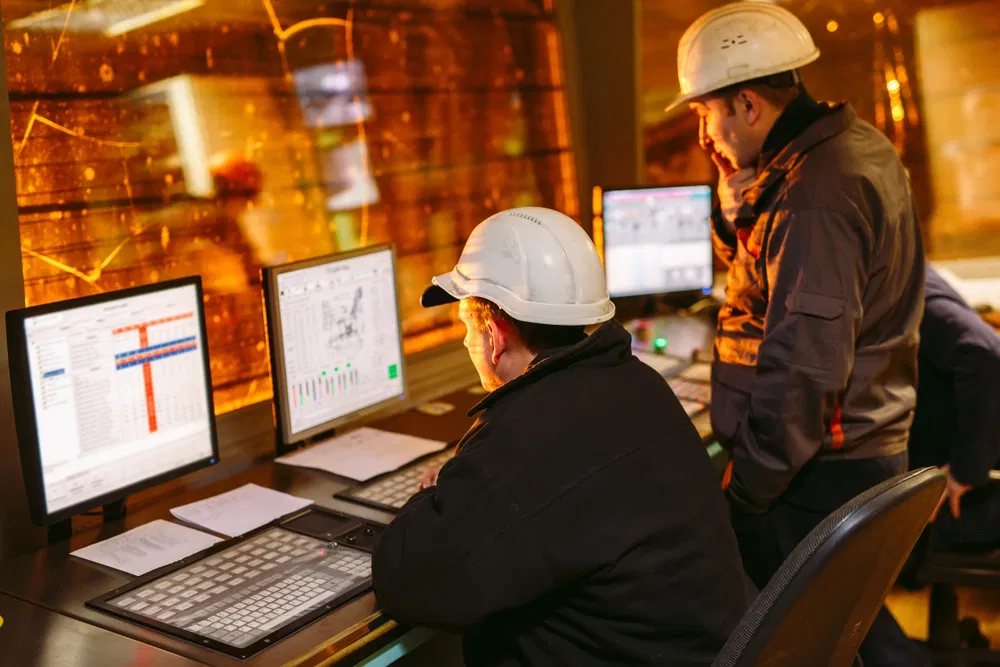
Every heavy industrial process must be monitoring all its parameters such as temperature, pressure, volumetric flow, concentration, etc. For some cases, like oil refineries, are very easy to calculate these parameters without measuring.
However, in most cases, this is not an easy task. In industries like biotech-pharmaceuticals, food, steel, plastics, and many others, is very difficult to create physicochemical models able to calculate process parameters.
Therefore, Artificial Intelligence and Deep Learning techniques come to do that: Estimate process parameters based on historical data working as virtual sensors.
For operators and engineers being able to know a parameter without actual measuring is very powerful, they can work with confidence when a physical sensor fails, or they can do a virtual measurement where a physical sensor is not present.
Some companies offering this product are UPTAKE, Arundo Analytics, McKinsey Analytics, Aspen Technology , and others.
2.4 Operation Knowledge Standardization
In order to have a virtual sensor you have to train your models with all the operational variables, but imagine adding other features like specific operator experience and feelings of the process, in other words, it’s to put human knowledge in the model.
Therefore, this approach allows us to have a better understanding of the process and facilitates the generational change that could be hard especially to complex processes like cement, refining, or big scale manufacturing.
2.5 Leakage Detection

Up to this point, I have shown to you heavy industries applications of AI using tabular data, it’s time to talk a little bit about computer vision.
Spills are a common problem that can occur in any industry that processes liquids. If the plant is very large, there are several places where there isn’t human supervision. Here is where computer vision deep learning models can help the industry.
Therefore, installing unsupervised cameras is a relatively cheap investment, connect them to a system using a deep learning algorithm trained to detect liquid leakage, and send an alarm, is even easier.
However, the difficult part is to have a very good dataset to train the deep neural network. One of the reasons is that is mandatory to have images with the actual liquid that the company processes.
Companies like Landing.AI are working to overcome these difficulties and offer good models to the industry.
2.6 Engineering Documents Digitization

Before its construction, every heavy industry process plant must execute all the engineering process, that consists of three global steps:
- Conceptual Engineering
- Basic Engineering
- Detail Engineering
During these steps, engineers generate lots of documents like diagrams, equipment lists, instrumentation lists, drawings, and many others. These documents are mandatory to have all the information necessary to build the designed facilities by the construction contractor.
Sometimes it is necessary to make changes or improvements to an already working plant, and generally, the documents are not complete or even not digitalized, these kinds of projects are named Brownfield projects.
So, the heavy industries companies contract an engineering firm to digitalize the documents, then the engineering firm contracts lots of junior engineers to do the task manually, spending a lot of money and time in a task that can be made using computer vision.
It could sound trivial but a tool to optimized these tasks is highly valuable at engineering firms.
Therefore, there are companies offering tools to digitalized process and instrumentation diagrams (P&IDs) using computer vision with deep learning models very well trained and tuned to detect symbols and text, the data already extracted could be exported to PDF or CSV to be processed in other software.
Arundo Analytics has developed a tool to digitalized P&IDs known as DataSeer.
These are some examples of how AI could be used to improve heavy industrial operations. So you can have a wider understanding of the real-world uses of this amazing technology.
I hope you enjoyed this article, my intention was to show you applications of Machine Learning different from what you usually hear.
Please, don’t hesitate to send me your feedback.